Modern computer vision heavily relies on deep learning, an approach that maps data to desired outputs. While deep learning has revolutionized object recognition and classification, it is important to explore the broader landscape of vision engineering.
At Mujin, we embrace a multifaceted approach that goes beyond the limitations of deep learning algorithms. In this article, we will delve into the challenges of doing computer vision for industrial robotics and highlight Mujin’s unique approach to achieving higher quality and tailored solutions compared to solely relying on deep learning.
Limitations of Deep Learning in industrial robotics
Deep learning excels at recognizing objects and making accurate predictions based on training data. However, when it comes to industrial robotics, there are additional complexities that demand a more nuanced understanding of the environment. Simply detecting objects is not sufficient; factors like positioning, context, and potential damage must be taken into account. Meaning that the variation of appearance is very high that cannot be included in the training data. Deep learning algorithms alone struggle to comprehend and address these intricacies, highlighting the need for a more intelligent and tailored approach.
Mujin’s thoughtful algorithms for real-world needs
At Mujin, we prioritize designing algorithms that solve real-world problems in industrial robotics. Our approach goes beyond object recognition, taking into consideration the specific needs and challenges of our customers.
For example, online order fulfillment involves intricate processes that go beyond simple object recognition. Customers expect their orders to be handled with care, ensuring that the received products are in pristine condition. This includes considerations such as avoiding damage such as wrinkling, maintaining the integrity of delicate items, and providing accurate packaging. Deep learning algorithms alone struggle to account for these complexities, necessitating a more sophisticated and nuanced approach.
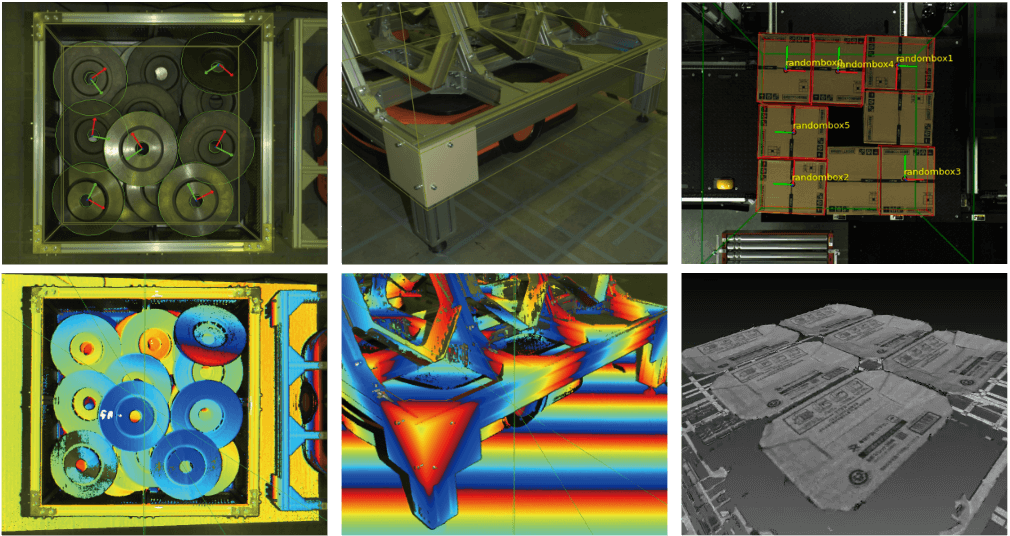
Our approach addresses the specific needs of each customer. We design algorithms that consider factors such as handling delicate items without causing damage, ensuring accurate packaging, and optimizing the entire fulfillment process for efficiency. At Mujin, we create tailored solutions that enhance the end user’s satisfaction.
Achieving superior quality and efficiency
While well trained deep learning models typically achieve performance rates between 95% and 98%, they may fall short in high-throughput scenarios. This level of performance may seem impressive, but it is crucial to recognize the importance of pushing beyond that threshold. At Mujin, we pride ourselves on going the extra mile and achieving the last 5% – the most challenging part of the journey. For customers handling thousands of items a day, even a small percentage of errors can have a significant impact.
Mujin’s unique approach allows us to achieve higher-quality outputs, minimize errors, optimize productivity, and ensure customer satisfaction surpassing the limitations of traditional deep-learning models.
Mujin’s commitment to achieving the last 5% stems from our belief that true excellence lies in meticulous attention to detail. While attaining the first 95% of accuracy may be relatively straightforward, it is the pursuit of perfection that truly sets us apart. We understand that in the world of robotics, even a single mistake can have far-reaching consequences. Just as a single failure jeopardizes a 25-year mission to the moon, we strive to ensure that our systems operate flawlessly and really have a performance of more than 99.99%.
Beyond data tuning: The Art of Algorithm Development
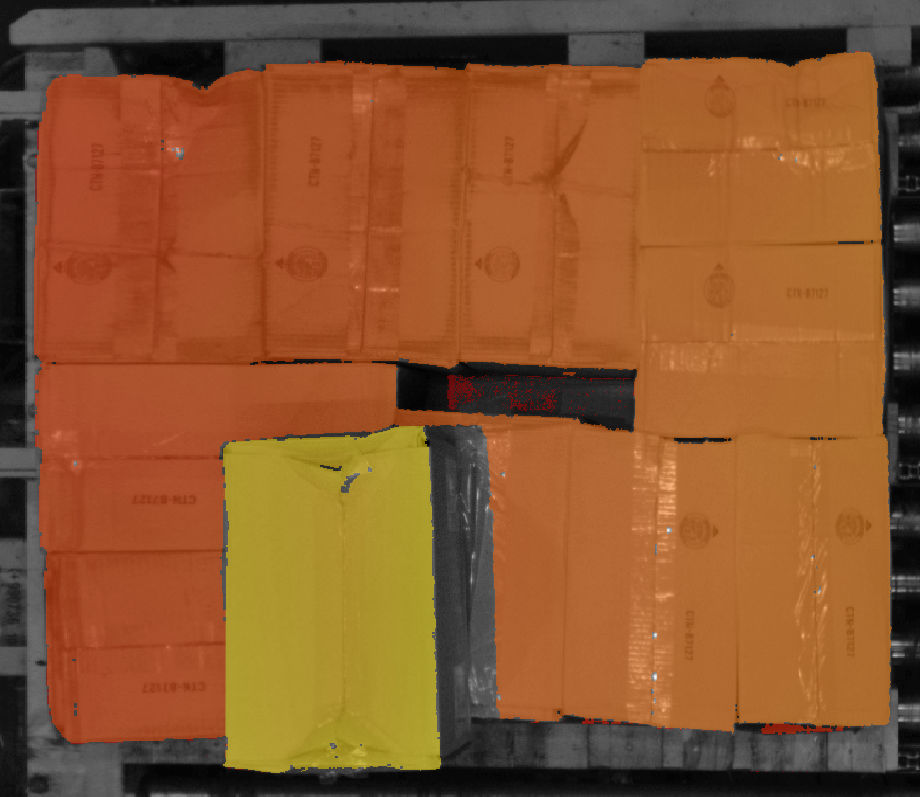
Deep learning often involves repetitive and tedious tasks such as obtaining a lot of data, labeling and model tuning, where parameters are adjusted, and models are retrained. However, at Mujin, we believe in going beyond this process, because achieving the last mile is not done by fine-tuning data or parameters, but in better understanding of the problem and the physical aspects of it. Our vision engineers analyze what properties can be used for robust detection and what will disturb these properties. In current deep learning networks, these properties are hard to embed and is only achieved by adding more data.
For example, understanding how a box is different when a corner is dented can provide insight on how to create an algorithm to detect that. No customer will be happy to hear that in order to create a dataset for training a few hundred of their items have to be broken.
With this intellectually stimulating approach, we continuously push the boundaries of what is possible in vision engineering.
Deep Learning Automation?
Looking to the future, we envision a world where deep learning has grown from a manual process that is considered a “black box”, to something that can be automatically adjusted. Adding features to a network, the need to manually create labelled datasets, and tune parameters should be a thing of the past. In doing so we can enhance scalability and efficiency in vision engineering, to ensure robotics is deployable in scale. This automation will free up human experts from repetitive tasks, allowing them to focus on higher-level responsibilities that require creativity and expertise. With automated deep learning, we can unlock new realms of innovation and revolutionize the field of computer vision.
If you are interested in being a part of the Computer Vision team, please apply directly through our career website.